Over the last 15 years, more than 1,000 people have been killed by volcanoes and associated hazards. Exploring and developing new ways to forecast volcanic eruptions near populated areas can help prevent more loss of human life. A new study led by Leoncio Cabrera from the Universidad de Chile compares geodetic data from GNSS and InSAR with a machine learning forecasting model to assess how well they can detect the precursors to eruptions.
Currently, a system called “volcano alert level” is used to indicate whether a volcano has a significant chance of erupting. This system is based on changes in activity, such as more frequent earthquakes associated with magma movement and off-gassing of SO2. The system can’t give a probability of eruption within a given timeframe, which ultimately makes coordination of evacuation and mitigation efforts difficult.
Typical forecasting methods have used a combination of seismic and geodetic data for short-term forecasting. However, it’s impossible to determine months or years in advance when a volcano may become a significant threat. Training a machine learning algorithm on seismic and data can help uncover short-term trends associated with eruption. Marrying this insight to gradual, subtle trends in volcano data recorded by geodetic technologies like GNSS and InSAR could help increase the reliability of forecasting.
In the case of volcanoes, it may be difficult to manually identify trends in geophysical metrics across different eruptions, even at the same volcano. So, a technique like machine learning could help. Machine learning is touted as a data-driven method with high adaptability best applied to systems where complex patterns and behavior are hard to detect otherwise. The team led by Cabrera set out to investigate how machine learning may be useful for predicting pre-eruption signals. They applied machine learning to data at the Copahue volcano in the Southern Andes in Chile, which has erupted six times between March 2020 and November 2021. Cabrera and fellow researchers wanted to determine if their model could forecast the eruptive episodes at Copahue based on behavioral precursors identified from previous eruptions.
To do this, machine learning tries to classify whether or not anomalies in the seismic and geodetic data (i.e., earthquakes associated with magma chamber movement and surface deformation) are significant predictors to an imminent eruption.
The team found that their machine learning model was able to correctly produce a warning for imminent eruption in five out of six eruptions. Even so, the successfully forecasted eruptions were not all predicted with the same lead time. Some alerts were issued within 5 hours of an eruption, while others had as many as 75 hours of forewarning. This points to the possibility that, even at the same volcano, pre-eruption behaviors in seismic and geodetic data are not the same between different eruptions.
While the machine learning method is overall successful, the differences in forecasting quality mean that this strategy is not yet ready to be implemented in real-life applications. Moreover, geodetic techniques are better for monitoring years-long cycles of volcanic behavior, while seismic techniques reveal activity over shorter time scales. Since this model was only trained on seismic data, its success reflects the ability to forecast the short-term activity seen in seismic records. Ultimately, seismic and geodetic data can elucidate different processes important to eruption forecasting, but the model is only thus far suitable for shorter term seismic data.
The researchers are very careful to point out the limitations of their method. Their machine learning model is specifically optimized for the type of eruptions that are common at Copahue—short-lived and driven by a combination of steam and magma—and so is not suitable for widespread application to other volcanoes and types of eruptions. But incorporating more types of data, such as gas emission measurements, infrasound, and seismicity rate together with the preexisting seismic and geodetic data could help the model work more broadly. Researchers at other volcano observatories can apply this method to improve the applicability.
At the end of the day, new techniques to study when, where, and how eruptions are likely to occur are exciting and open up opportunities for technological improvement, but forecasting tools must be carefully implemented. A model is not infallible and is only as good as the data it’s fed.
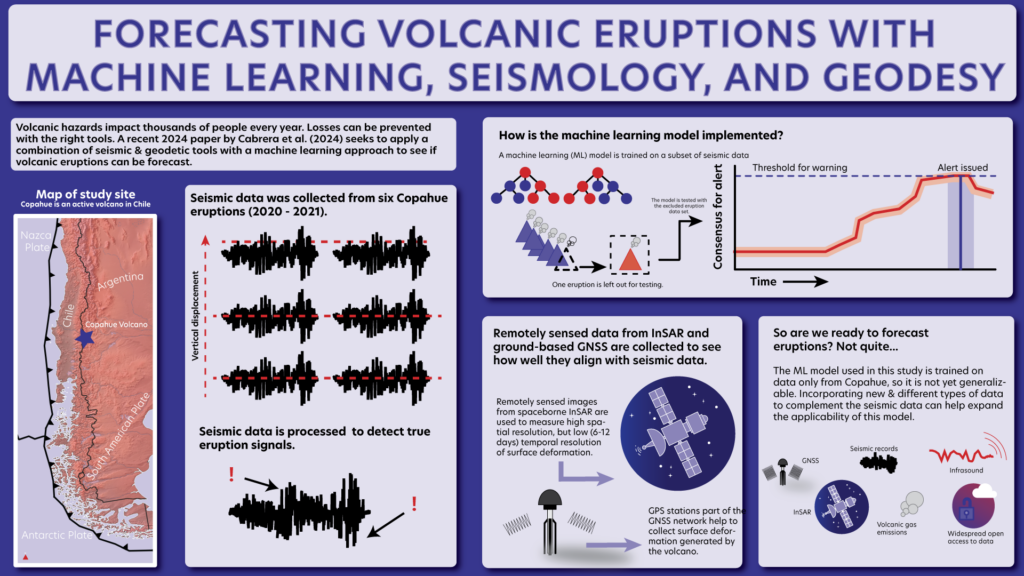