The EarthScope-operated data systems of the NSF GAGE and SAGE Facilities are migrating to cloud services. To learn more about this effort and find resources, visit earthscope.org/data/cloud
Distributed Acoustic Sensing (DAS) opens new scientific doors because of its unique capabilities, but getting through those doors can be a bit of a logistical challenge. The tremendous amount of data generated can seem to fill hard drives faster than you can say “there are one thousand gigabytes in a terabyte”, putting the data processing beyond the means of the humble personal computer.
But with the right workflow, this can all become tractable, allowing researchers to focus on the interesting signals in DAS data. A recent paper in the Journal of Glaciology used DAS on a 9 km fiber cable deployed along the centerline of Rhône Glacier in the Swiss Alps to infer the discharge of meltwater streams. The study compares three machine learning methods applied to a month of data, finding an effective data processing technique to pull out that record of meltwater discharge.
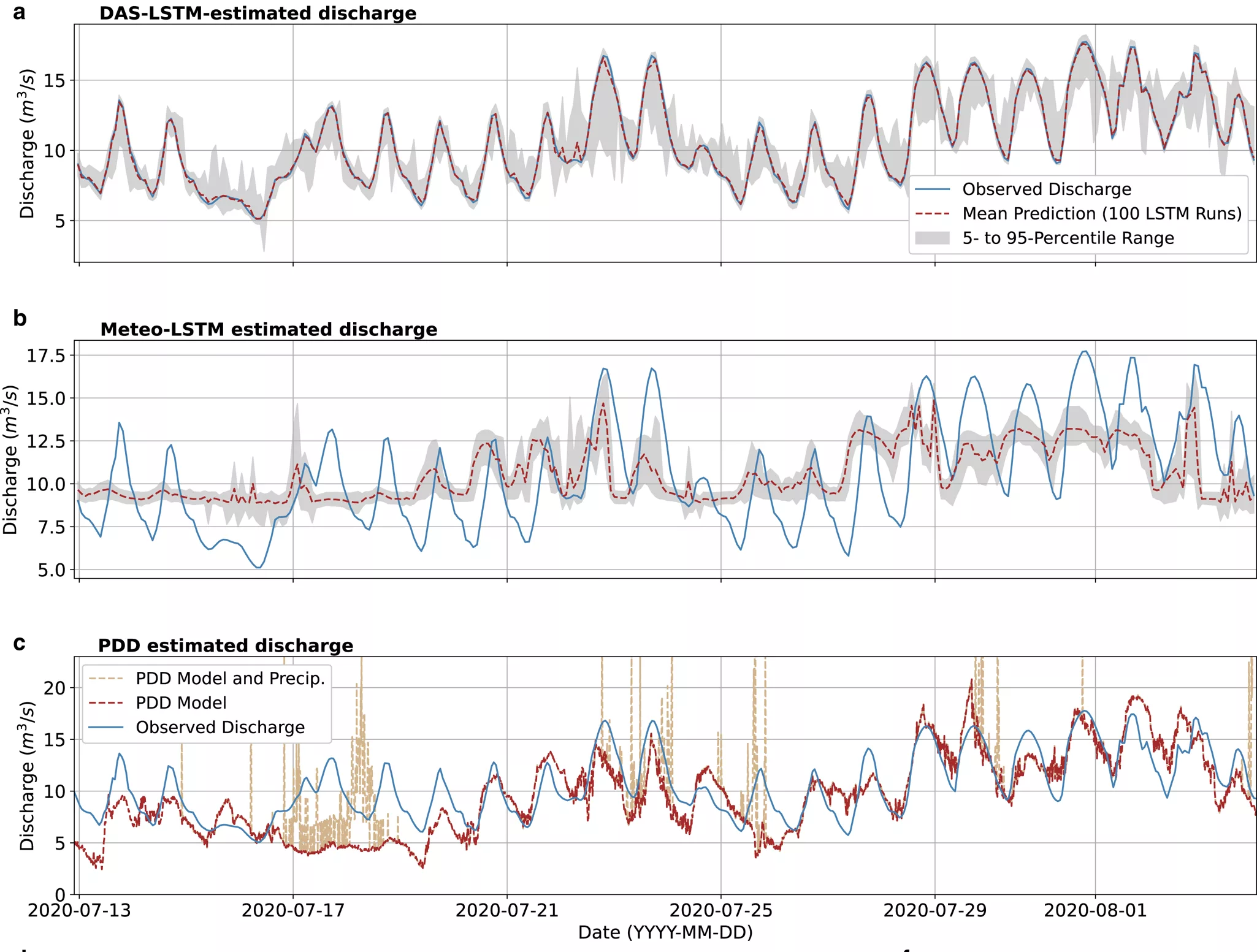
We sat down with first author John-Morgan Manos to talk through the behind-the-scenes data processing work required for this project. Listen in to find out how they took 20 terabytes of data recorded in the Alps and put it through multiple machine learning workflows—and what developments on the horizon could simplify this work in the future.